Artificial Intelligence (AI) is revolutionizing various industries, and the financial sector is no exception. AI financial analysis is transforming investment strategies, providing unprecedented insights and efficiencies. This article explores the profound impact of AI financial analysis on investment strategies, highlighting key innovations, applications, and future trends.
Introduction to AI Financial Analysis
Understanding AI in Finance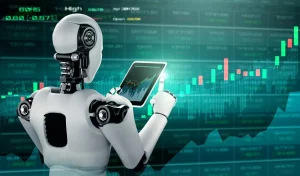
AI financial analysis involves using artificial intelligence technologies to analyze financial data, predict market trends, and optimize investment strategies. These technologies include machine learning, natural language processing (NLP), and deep learning, which process vast amounts of data to generate actionable insights.
Evolution of AI Financial Analysis
The evolution of AI financial analysis has been marked by significant advancements in computational power, data availability, and algorithmic sophistication. Early applications focused on automating routine tasks, but today’s AI systems can perform complex analyses, identify patterns, and make predictions with remarkable accuracy.
Key Innovations in AI Financial Analysis
Machine Learning Models
Machine learning models are at the core of AI financial analysis. These models learn from historical data to predict future market trends and asset prices. Techniques such as regression analysis, decision trees, and neural networks are commonly used to develop predictive models.
Regression Analysis
Regression analysis helps in understanding the relationship between different financial variables and predicting future values. It is widely used in stock price forecasting and risk assessment.
Neural Networks
Neural networks mimic the human brain’s structure to process complex data. They are particularly effective in recognizing patterns and making predictions, making them valuable tools in financial analysis.
Natural Language Processing (NLP)
NLP enables AI systems to analyze textual data from financial reports, news articles, and social media. By understanding sentiment and extracting relevant information, NLP enhances decision-making in investment strategies.
Sentiment Analysis
Sentiment analysis uses NLP to gauge market sentiment by analyzing textual data. It helps investors understand public perception and potential market reactions to news and events.
Deep Learning
Deep learning, a subset of machine learning, involves neural networks with multiple layers. It excels in handling large datasets and complex patterns, making it ideal for financial analysis.
Convolutional Neural Networks (CNNs)
CNNs are used for image and pattern recognition, while in finance, they can analyze market charts and identify trading signals.
Recurrent Neural Networks (RNNs)
RNNs are effective in processing sequential data, such as time-series data in stock prices, making them valuable for predicting market trends.
Applications of AI Financial Analysis
Predictive Analytics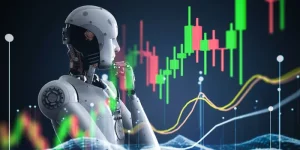
Predictive analytics is one of the most significant applications of AI financial analysis. It involves using historical data to forecast future market trends and asset prices, helping investors make informed decisions.
Stock Price Forecasting
AI models analyze historical stock prices and market indicators to predict future prices. This enables investors to time their trades and optimize returns.
Risk Assessment
AI financial analysis assesses risks by analyzing market volatility, economic indicators, and historical data. It helps investors manage risks and protect their portfolios.
Portfolio Management
AI financial analysis optimizes portfolio management by providing personalized investment strategies. It considers factors such as risk tolerance, investment goals, and market conditions to create balanced portfolios.
Robo-Advisors
Robo-advisors are AI-driven platforms that offer automated investment advice and portfolio management. They use algorithms to provide personalized recommendations and manage investments.
Algorithmic Trading
Algorithmic trading uses AI algorithms to execute trades based on predefined criteria. It enhances trading efficiency and accuracy, allowing investors to capitalize on market opportunities.
High-Frequency Trading (HFT)
HFT is a form of algorithmic trading that executes trades at high speeds and volumes. AI algorithms identify minor price discrepancies and execute trades within milliseconds.
Fraud Detection
AI financial analysis enhances fraud detection by analyzing transaction data and identifying suspicious activities. Machine learning models detect anomalies and patterns indicative of fraudulent behavior.
Anomaly Detection
Anomaly detection algorithms identify unusual patterns in financial data, signaling potential fraud. They enhance security and protect against financial crimes.
Case Studies of AI Financial Analysis
JPMorgan Chase
JPMorgan Chase uses AI financial analysis to enhance its trading operations. The bank’s AI-driven platform, LOXM, executes trades with high precision and efficiency, optimizing trading strategies.
BlackRock
BlackRock, the world’s largest asset manager, leverages AI to optimize portfolio management. The firm’s Aladdin platform uses AI algorithms to analyze market trends, assess risks, and develop personalized investment strategies.
Renaissance Technologies
Renaissance Technologies is renowned for its use of AI in trading. The firm’s Medallion Fund employs complex AI algorithms to analyze market data and execute trades, demonstrating the transformative power of AI financial analysis.
Future Trends in AI Financial Analysis
Explainable AI
Explainable AI aims to make AI systems more transparent and interpretable. In financial analysis, explainable AI provides clear explanations for AI-driven predictions and decisions, enhancing trust and regulatory compliance.
Integration with Blockchain
Integrating AI with blockchain technology offers significant advantages in terms of security, transparency, and efficiency. Blockchain provides a secure and immutable ledger for transactions, while AI analyzes blockchain data to detect patterns and anomalies.
Quantum Computing
Quantum computing holds the potential to revolutionize AI financial analysis by offering unprecedented computational power. Quantum computers can process complex calculations much faster than classical computers, enhancing predictive analytics and risk management.
Personalized Financial Services
AI is paving the way for personalized financial services, offering tailored solutions based on individual preferences and needs. AI-driven platforms provide customized investment recommendations, financial planning advice, and credit scoring.
Ethical and Responsible AI
Ensuring ethical and responsible AI practices is crucial for maintaining trust and integrity in financial analysis. Financial institutions must implement guidelines and frameworks to promote fairness, transparency, and accountability in AI systems.
Implementing AI Financial Analysis: Best Practices
Ensuring Data Quality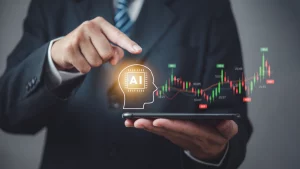
Data quality is critical for the success of AI financial analysis. Financial institutions should invest in robust data management practices to ensure the accuracy, completeness, and consistency of their data.
Continuous Learning and Development
Continuous learning is essential for staying ahead of the curve in financial analysis. Financial institutions should invest in ongoing training and development for their AI teams, keeping them updated on the latest advancements and best practices.
Collaboration and Cross-Disciplinary Teams
Successful implementation of AI financial analysis requires collaboration between data scientists, financial analysts, and regulatory experts. Cross-disciplinary teams bring diverse perspectives and expertise, enhancing the development and implementation of AI solutions.
Ethical AI Practices
Ensuring ethical AI practices is crucial for maintaining trust and integrity in financial analysis. Financial institutions should implement guidelines and frameworks to promote fairness, transparency, and accountability in AI systems.
Investing in Security
The security of AI systems is paramount, especially in the financial industry. Financial institutions should implement robust security measures to protect AI models and data from cyber threats.
Conclusion
The integration of AI financial analysis is revolutionizing investment strategies, offering unprecedented insights, efficiency, and accuracy. From predictive analytics and portfolio management to algorithmic trading and fraud detection, AI is transforming financial practices and driving better decision-making.
By understanding and embracing these advancements, financial institutions can unlock new opportunities, optimize their operations, and achieve greater success. Implementing best practices, including ensuring data quality, continuous learning, collaboration, ethical AI practices, and investing in security, is essential for maximizing the benefits of AI financial analysis
Stock Exchange AI is Transforming Modern Trading
Artificial Intelligence and Stock Exchange: Best Revolutions